torchaudio.transforms
torchaudio.transforms
模块包含了常见的音频处理和特征提取功能。下图展示了一些可用变换之间的关系。
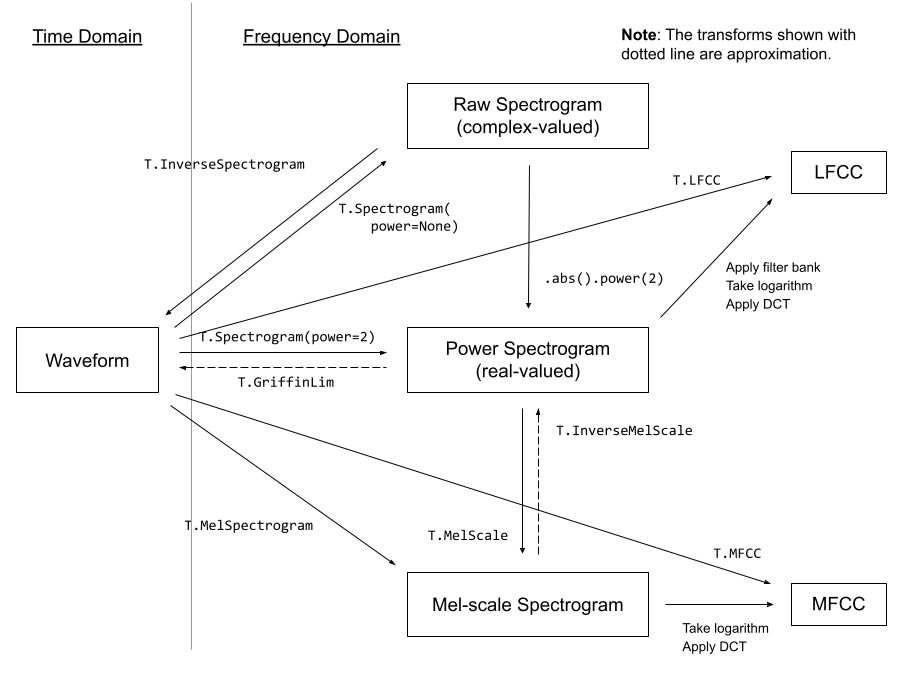
这些变换是通过 torch.nn.Module
实现的。构建处理管道的常见方法是定义自定义的 Module 类,或者使用 torch.nn.Sequential
将多个 Module 串联起来,然后将其移动到目标设备和数据类型。
# Define custom feature extraction pipeline.
#
# 1. Resample audio
# 2. Convert to power spectrogram
# 3. Apply augmentations
# 4. Convert to mel-scale
#
class MyPipeline(torch.nn.Module):
def __init__(
self,
input_freq=16000,
resample_freq=8000,
n_fft=1024,
n_mel=256,
stretch_factor=0.8,
):
super().__init__()
self.resample = Resample(orig_freq=input_freq, new_freq=resample_freq)
self.spec = Spectrogram(n_fft=n_fft, power=2)
self.spec_aug = torch.nn.Sequential(
TimeStretch(stretch_factor, fixed_rate=True),
FrequencyMasking(freq_mask_param=80),
TimeMasking(time_mask_param=80),
)
self.mel_scale = MelScale(
n_mels=n_mel, sample_rate=resample_freq, n_stft=n_fft // 2 + 1)
def forward(self, waveform: torch.Tensor) -> torch.Tensor:
# Resample the input
resampled = self.resample(waveform)
# Convert to power spectrogram
spec = self.spec(resampled)
# Apply SpecAugment
spec = self.spec_aug(spec)
# Convert to mel-scale
mel = self.mel_scale(spec)
return mel
# Instantiate a pipeline
pipeline = MyPipeline()
# Move the computation graph to CUDA
pipeline.to(device=torch.device("cuda"), dtype=torch.float32)
# Perform the transform
features = pipeline(waveform)
请查看涵盖 transforms 深入使用教程。
实用工具
特征提取
数据增强
以下变换实现了被称为 SpecAugment 的流行数据增强技术 [Park et al., 2019]。
损失
多通道
|
|
PSD |
计算跨通道功率谱密度 (PSD) 矩阵。 |
MVDR |
最小方差无失真响应 (MVDR) 模块,用于执行基于时频掩码的 MVDR 波束成形。 |
RTFMVDR |
最小方差无失真响应 (Minimum Variance Distortionless Response)MVDR[Capon, 1969基于噪声的相对传递函数(RTF)和功率谱密度(PSD)矩阵的模块。 |
SoudenMVDR |
最小方差无失真响应 (Minimum Variance Distortionless Response)MVDR[Capon, 1969]) 基于所提出方法的模块Souden 等人[Souden等人, 2009]. |